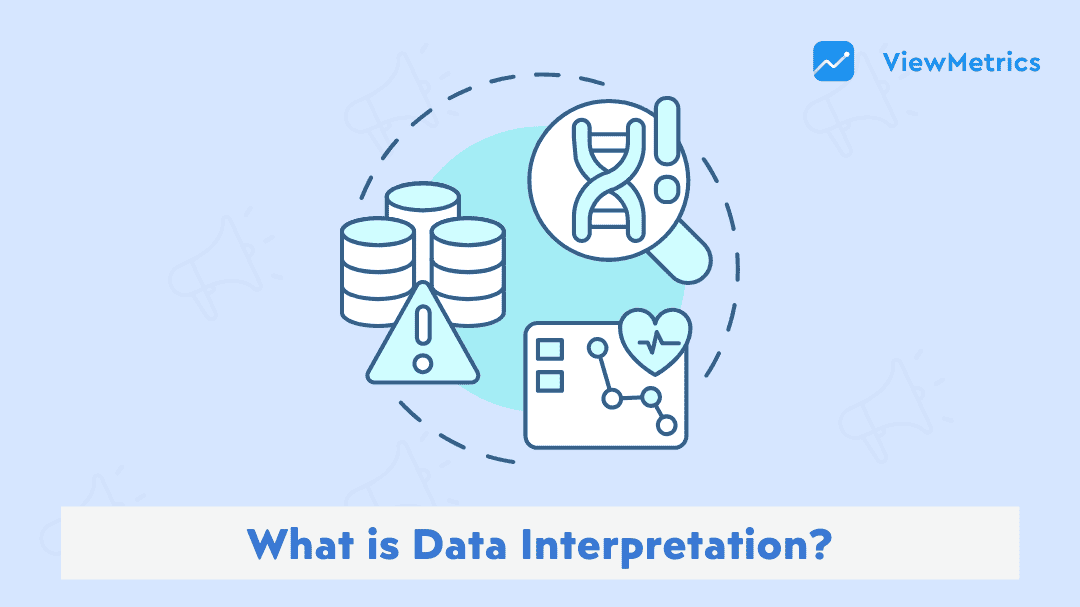
Effective data interpretation is crucial in today’s data-enabled world. For an organization to thrive, it transforms complex information into meaningful insights, enabling businesses to identify trends and make informed decisions.
But what is data interpretation? What are its types, and what is the method of interpreting data? Keep reading to learn more.
What is Data Interpretation?
Interpreting data is all about taking a close look at information and drawing meaningful conclusions by using a range of analytical methods. It helps researchers tweak, sort, and summarize data to uncover answers to important questions.
In the business world, data interpretation is more than just number-crunching—it’s the process of examining and refining data to spot patterns, trends, and behaviors. As a data manager, these insights allow you to make smart, well-informed decisions based on solid evidence, ensuring you have all the facts in front of you.
Note: Before interpreting data, you must check this guide on how to track data and what data tracking is.
What are the Types of Data Interpretation?
There are three types of data interpretation, including:
1. Quantitative Data Interpretation
Quantitative data interpretation involves analyzing numbers to make sense of them. This type of data is typically used to measure and evaluate specific metrics, like sales performance, customer satisfaction scores, or employee productivity levels. It’s all about turning raw numbers into valuable insights.
2. Qualitative Data Interpretation
Qualitative data interpretation focuses on analyzing non-numerical information like text, images, or audio. It’s commonly used to explore customer opinions and attitudes, helping you uncover deeper insights and spot patterns or trends that might not be immediately obvious from numbers alone.
3. Mixed Methods Data Interpretation
Mixed methods data interpretation blends both quantitative and qualitative data to offer a more well-rounded understanding of a topic.
This approach is especially helpful when dealing with data that includes numbers and non-numerical insights, like customer feedback, allowing for a fuller picture of the situation.
What is the Process of Interpreting Data?
Now that you know the types of data interpretation, it’s time to learn how to interpret data, from beginning to the ending.
There are six key steps in data interpretation, including baseline establishment, data collection, data preparation, interpretation, visualization, and reflection. Let’s learn about them in detail:
1. Baseline Establishment
Just like in a competitive analysis, the first thing you need to do when interpreting data is establish a clear starting point. This means setting specific objectives and mapping out both your short-term and long-term goals that could be influenced by what you find in the data.
For instance, if you’re an investor, you might focus on setting targets related to the return on investment (ROI) for the companies you’re assessing. Don’t forget, at this stage, it’s equally crucial to decide which kind of data you’ll be diving into for analysis and interpretation.
2. Data Collection
Once you have established your baseline, it’s time to collect data. A good way to start is by visualizing it—may be through a bar chart, graph, or pie chart.
The goal here is to ensure you’re analyzing the data clearly and without any bias. At this point, think back to how you conducted your research.
Here are a couple of key questions to ask yourself:
- Did anything change or go wrong during the data collection process?
- Do you have any notes or observations saved from that time?
- Once you’ve gathered all your data and answered these questions, you’re ready to move on to the next step.
Related Read: What is Data Blending?
3. Data Preparation
After gathering your data, the next step is getting it ready for analysis. This often means cleaning it up by correcting any errors, filling in missing values, or removing outliers. You might also need to reformat the data to make it easier and more effective to analyze.
4. Interpretation
This step is crucial in the data interpretation process—it’s where you actually analyze the data you’ve gathered. This is also where you’ll decide whether to take a qualitative or quantitative approach.
If you choose qualitative analysis, you’ll be looking at the data from a more subjective angle. When using AI-driven tools for this, you’ll need to “code” the data extensively to capture personal sentiments that can’t be easily represented by numbers.
On the other hand, quantitative analysis involves a more objective, numbers-based approach. You’ll work with raw numerical data, calculating things like mean, standard deviation, and ratios. From there, you can use statistical models to dive deeper into behaviors, helping to predict trends and outcomes.
5. Visualization
Once your analysis is finished, it’s time to visualize your data and extract insights from different angles. Many companies today use dashboards for this step, offering quick, real-time insights through algorithms.
However, even without a dashboard, visualizing data is fairly simple. You’ll just need to format your data in a way that makes it easy to present visually.
Some common formats you can use include:
- Bar charts
- Tables
- Scatter plots
- Line graphs
- Pie charts
- Histograms
ViewMetrics provides an impressive array of customizable charts and graphs, allowing you to effortlessly create the ideal visual for your data. With its interactive dashboards, you can dive into your data from various perspectives and discover insights you might have otherwise overlooked.
6. Reflection
Finally, after you’ve crafted the right visualizations that align with your initial goals, it’s time to take a step back and reflect. While this might feel like a simpler task compared to the earlier stages, it’s actually a critical moment that can shape how effectively you interpret your data.
Use this time to review the entire data analysis process, search for any hidden relationships, and spot outliers or mistakes that might have slipped through during data cleaning. It’s important here to distinguish between correlation and causation, recognize any biases, and catch insights you may have overlooked.
Smarter Decisions with ViewMetrics – Get real-time insights, seamless integrations, and comprehensive reporting—all in one place.
How Can Data Interpretation Used in Different Industries?
There are several use cases for data interpretation in different industries and settings, such as marketing, healthcare, financial services, retail, and manufacturing.
Let’s see how data interpretation can be used in these different industries:
- Marketing – Marketers analyze customer behaviors, preferences, and trends through data to develop targeted strategies and campaigns.
- Finance – Financial companies evaluate investment performance through data, helping them make informed decisions on investment strategies.
- Healthcare – Healthcare professionals assess patient histories and test results using data, aiding in accurate diagnoses and treatment plans.
- Manufacturing – Manufacturers use data to monitor machine performance and inventory levels, improving production efficiency and management.
- Retail – Retailers study sales figures and customer habits to optimize merchandising and pricing strategies.
What are the Limitations of Interpreting Data?
There are some limitations to data interpretation, such as:
- The quality of data directly impacts the accuracy of interpretation—poor data leads to flawed conclusions.
- Cognitive biases and preconceptions can influence how data is interpreted, often leading us to see what we want or expect to see.
- Interpretation is subjective—what appears as a clear pattern to one person might seem like random noise to another.
- It can be time-consuming to properly interpret data.
- Communicating results in a way that others understand and agree with can be challenging.
- Specialized skills and knowledge are often required, and professional assistance may be necessary.
- External factors, like confounding variables, can affect the observed patterns and aren’t always accounted for in the data.
- Identifying patterns is inherently difficult because randomness exists in all data sets.
- Using multiple interpretation methods can help reduce bias and improve accuracy.
Data Analysis Vs. Data Interpretation
Aspects |
Data Analysis |
Data Interpretation |
---|---|---|
Objective |
The main goal is to process and organize raw data to uncover trends and patterns. |
Use the analyzed data to make sense of it, provide context, and draw conclusions. |
Process |
It starts with gathering data, then cleaning it up, transforming it into a usable format, and finally applying analytical methods to draw insights. |
It includes analyzing and interpreting the results to explain key insights and recommend the next steps. |
Focus |
It helps answer the ‘what’ and ‘how’ questions surrounding the data. |
It helps answer the ‘why’ and ‘what next’ aspects based on the results. |
Nature |
It is more quantitative and technical. |
It is more qualitative and subjective. |
Outcome |
Known to produce statistical outputs, structured data, and models. |
Known to provide more conclusions, insights, and recommendations. |
Decision-Making |
Provides evidence and data for supporting decisions. |
Influences decision-making by directly informing. |
Dependency | It can be done independently, but its effectiveness is restricted without proper interpretation. | Depends on the outcomes of data analysis and can’t happen without it. |
How to Create a Data Interpretation Report with ViewMetrics?
While data interpretation is necessary when analyzing your data and coming to conclusive decisions, it doesn’t need to be time-consuming. Creating manual data interpretation reports requires you to go through the tedious process of logging into multiple marketing tools, copying and pasting the data to a spreadsheet, and then repeating the same process for every client.
But with ViewMetrics, you can eliminate this old-school way by adopting the right amount of automation! All you have to do is connect all your marketing accounts in our platform, select a presentation template, and we’ll handle the rest.
Work smarter by automating client reports now
Get Started for free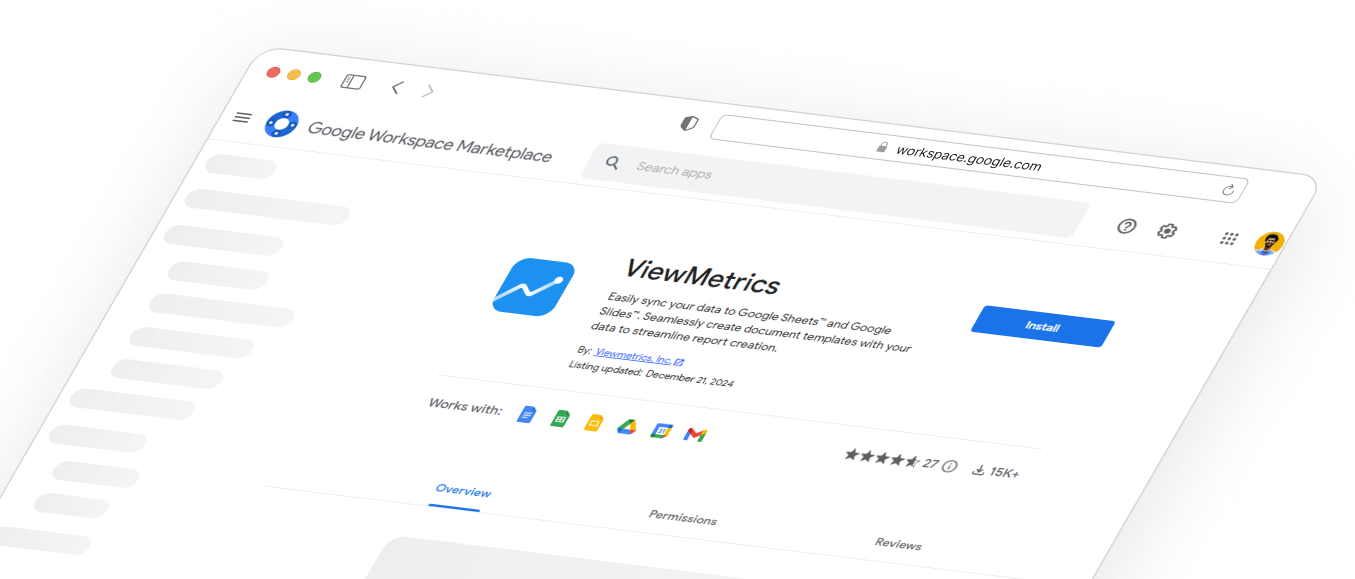

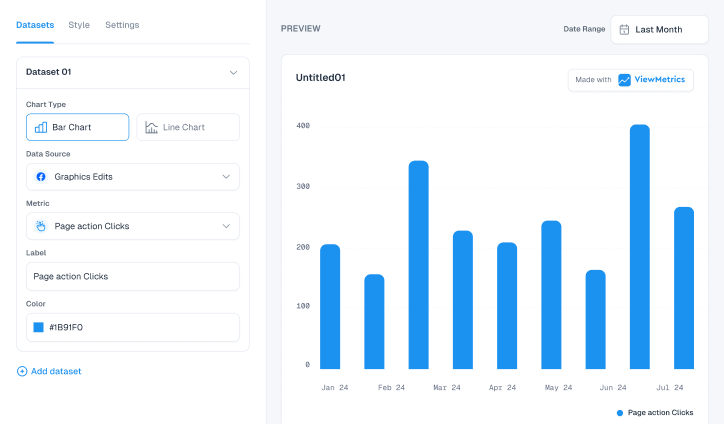
Design That Stands Out
Elevate visuals effortlessly with customizable styling options tailored to match brand aesthetics.

Effortless Data Syncing
Keep insights up-to-date with seamless and flexible data synchronization across platforms.
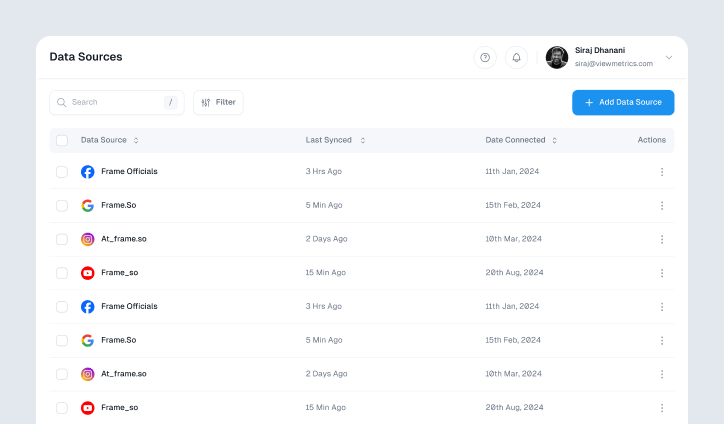
Connect with Ease
Integrate effortlessly with leading platforms to streamline workflows and maximize efficiency.
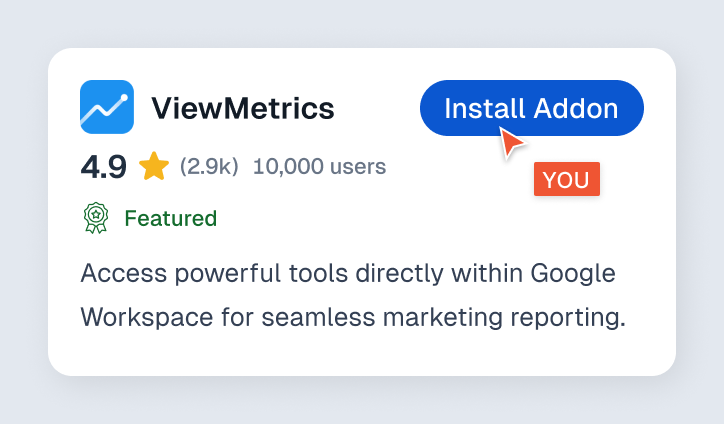
Boost Productivity with Google Add-On
Access powerful tools directly within Google Workspace for seamless marketing reporting.

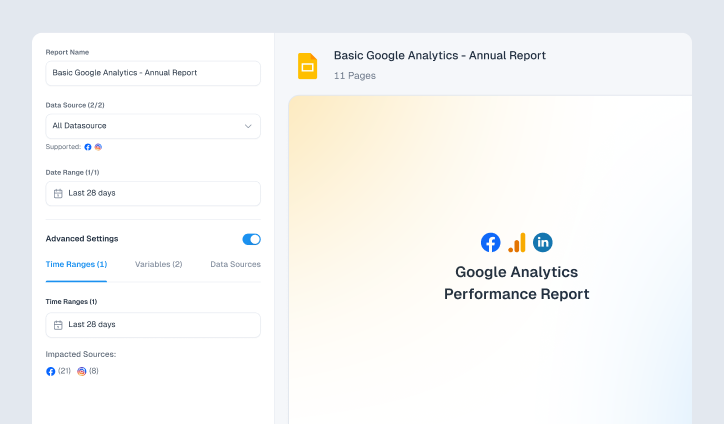
Custom Reports, Instant Insights
Generate tailored marketing reports effortlessly, delivering key insights in just a few clicks.

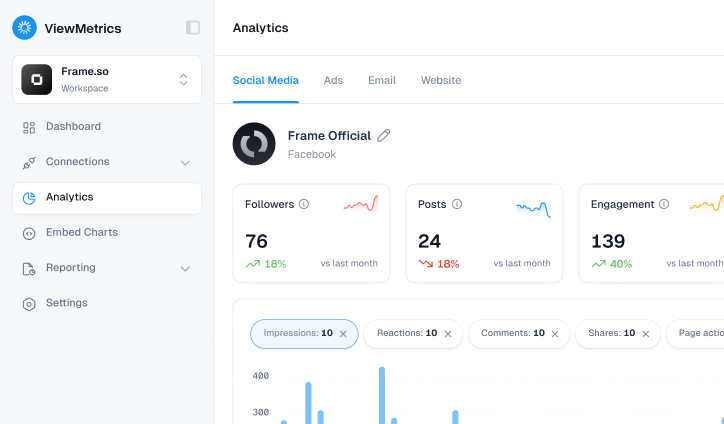
Actionable Analytics at a Glance
Access in-depth analytics to uncover trends, optimize strategies, and drive impactful decisions.

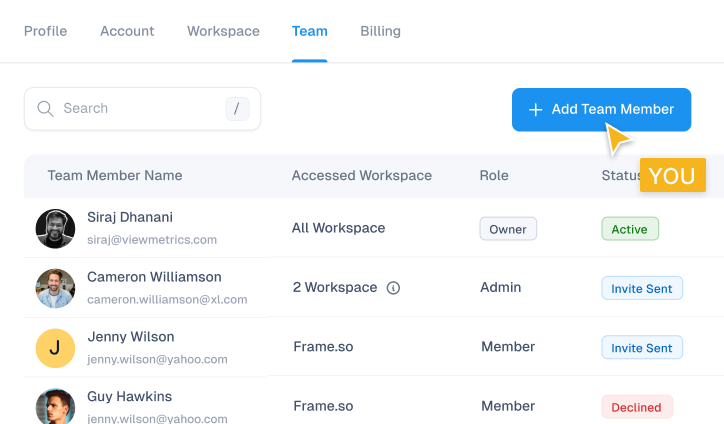
Collaborate with Ease
Invite team members to share insights, streamline workflows, and work together seamlessly.

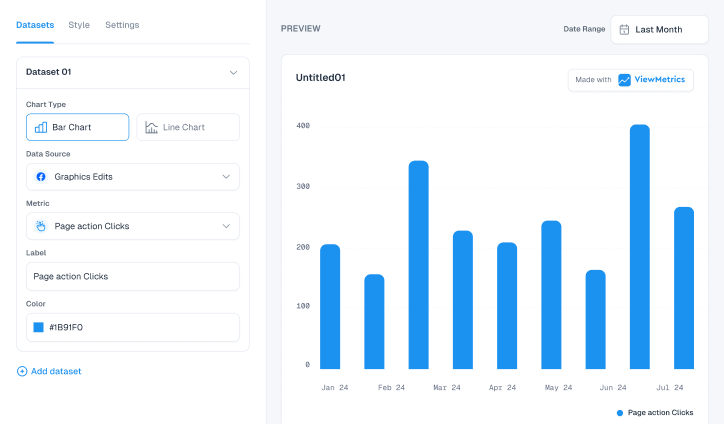
Design That Stands Out
Elevate visuals effortlessly with customizable styling options tailored to match brand aesthetics.

Effortless Data Syncing
Keep insights up-to-date with seamless and flexible data synchronization across platforms.
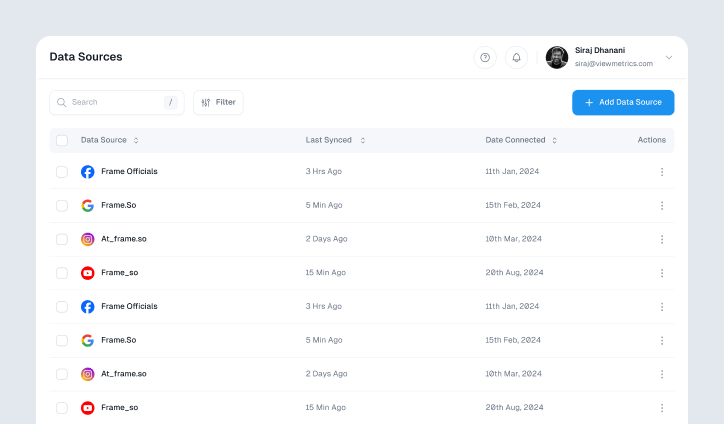
Connect with Ease
Integrate effortlessly with leading platforms to streamline workflows and maximize efficiency.
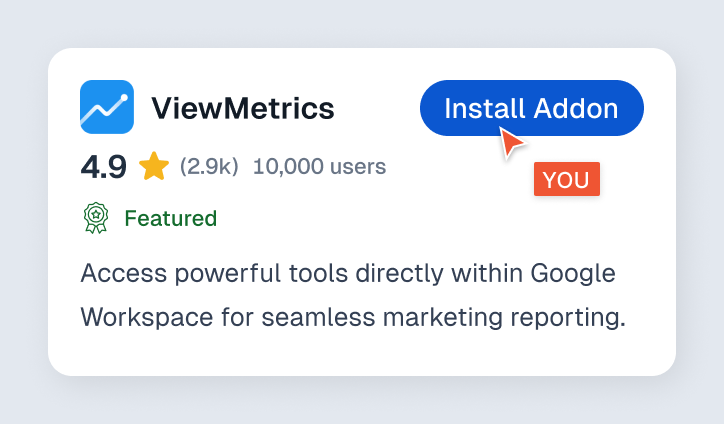
Boost Productivity with Google Add-On
Access powerful tools directly within Google Workspace for seamless marketing reporting.

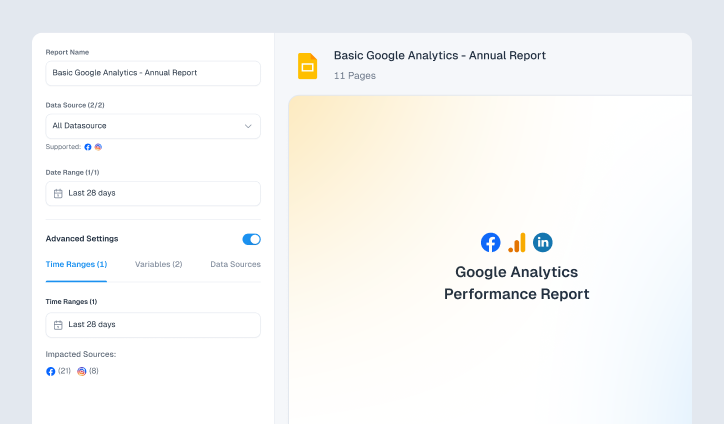
Custom Reports, Instant Insights
Generate tailored marketing reports effortlessly, delivering key insights in just a few clicks.

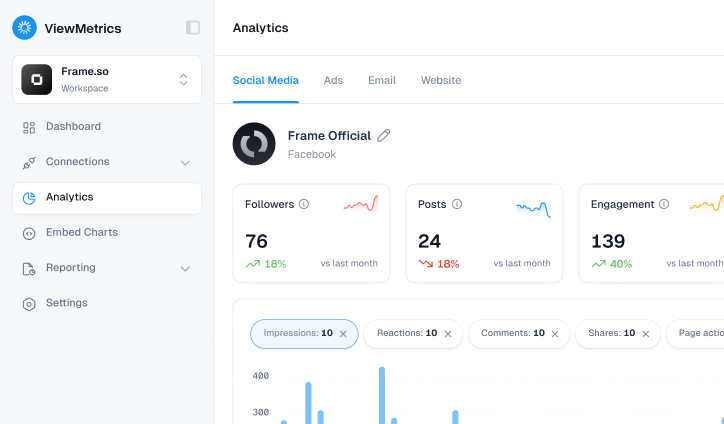
Actionable Analytics at a Glance
Access in-depth analytics to uncover trends, optimize strategies, and drive impactful decisions.

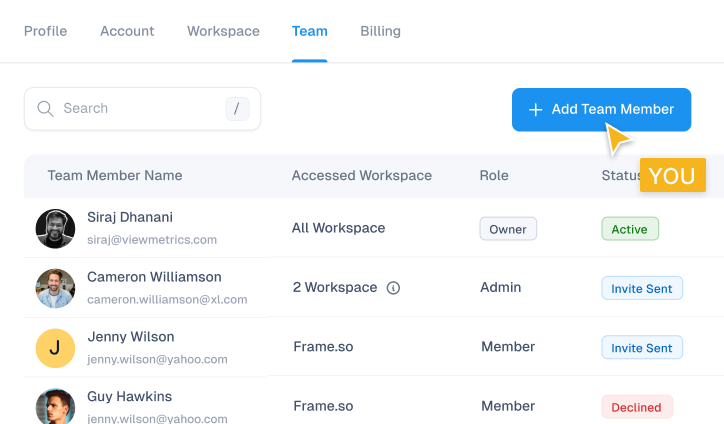
Collaborate with Ease
Invite team members to share insights, streamline workflows, and work together seamlessly.

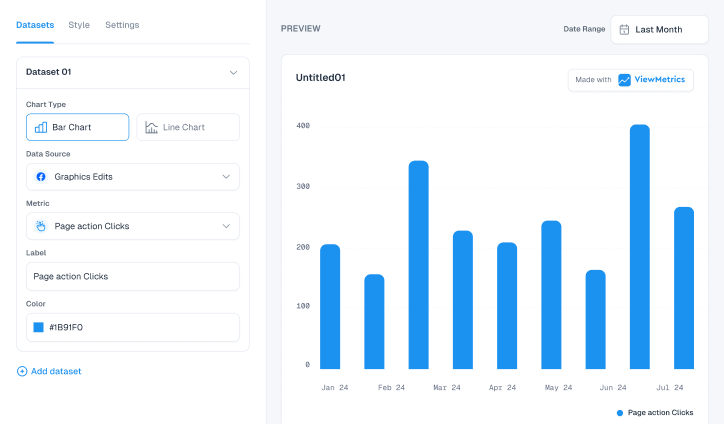
Design That Stands Out
Elevate visuals effortlessly with customizable styling options tailored to match brand aesthetics.

Effortless Data Syncing
Keep insights up-to-date with seamless and flexible data synchronization across platforms.
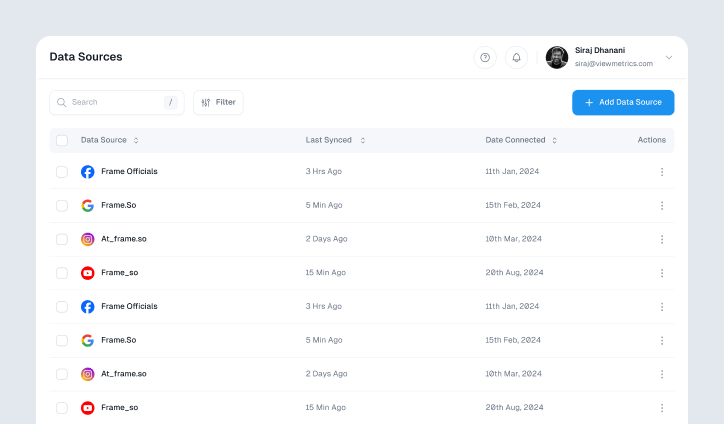
Connect with Ease
Integrate effortlessly with leading platforms to streamline workflows and maximize efficiency.
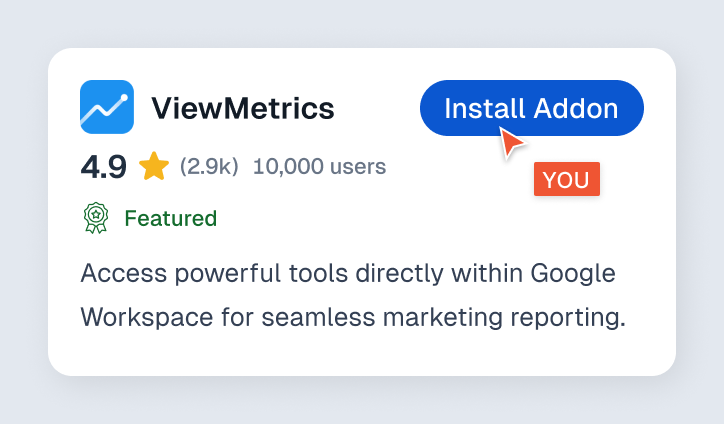
Boost Productivity with Google Add-On
Access powerful tools directly within Google Workspace for seamless marketing reporting.

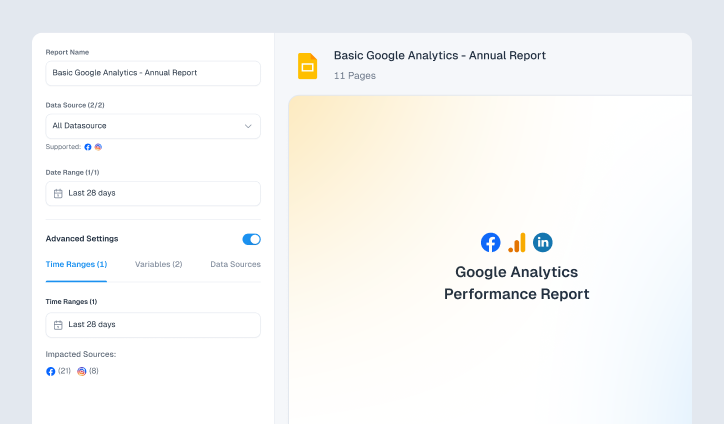
Custom Reports, Instant Insights
Generate tailored marketing reports effortlessly, delivering key insights in just a few clicks.

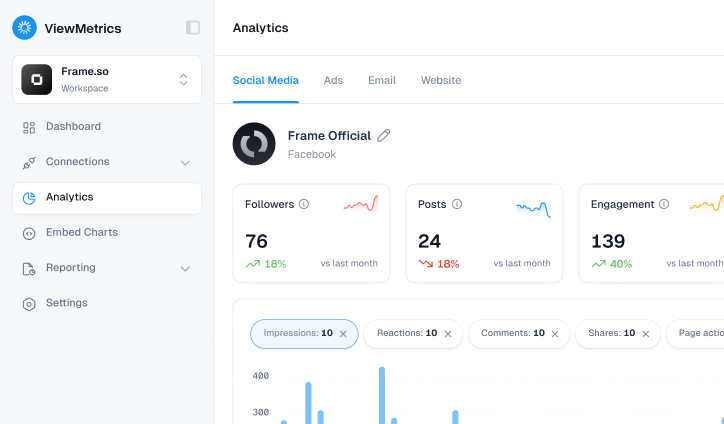
Actionable Analytics at a Glance
Access in-depth analytics to uncover trends, optimize strategies, and drive impactful decisions.

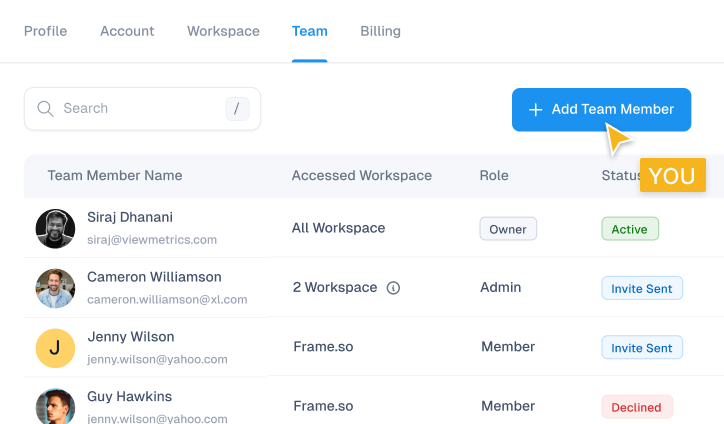
Collaborate with Ease
Invite team members to share insights, streamline workflows, and work together seamlessly.